Explore & argue with data
In the last blog post, we discussed data and information definitions.
We also touch on the concept level in the different data types—this time, we write about how and why it's critical for you to become a detective with your team.
The data projects are never as simple as they appear in the boardroom presentation. Stakeholders typically see a polished presentation that follows a rigid script from questions, to data, to answers.
What's lost in the story are all the old ideas that didn't make the it, important decisions, and the assumptions the data team made along the way to arrive at their answer.
Think about this: you bring a problem to resolve, build two teams, and provide the same exact data. These two teams might take separate analysis paths, possibly landing on the same conclusion or possibly not; there are just too many decisions to make along the way for any two teams, and each person will bring their background, ideas, and tools to make recommendations on how to best solve the problem therefore what is the most important rules in the exploratory data analysis is to ask the right questions and things to watch out as your team explores the data.
Smart people make mistakes. People and organizations can make mistakes.
As your team gets further along in the journey, the team circle back to earlier ideas, only to see multiple resolution paths. This process of iteration, discovery and that creativity is known as:
Exploratory data analysis [EDA]
EDA is an excellent practice to understand the data first and gather as many insights from it. EDA is all about making sense of data in hand before getting them dirty with it.
One of the most interesting things about EDA it's that there is no one right path to follow. EDA should not be thought of as a list of tools or a checklist.
The goal is to make sense of data with statistics and visualization before applying more complex methods. This is equivalent to detective work, clues are hidden in data, and exploration will reveal the next steps to follow.
Another key result of this process exploration is that while unsatisfying, EDA could stop you and your team from wasting your time and money on dead-end problems.
The success of a data strategy is how you make data types work together to create questions or provide answers, solve challenges and shift to data-driven decision-making. Create and reinvent new roles, positions, and duties that sharpen focus on data and analytics.
"Digital business transformation requires a new breed of data executives" - J.C.
New and advanced analytics technologies are on the rise, such as predictive and prescriptive analytics, decision management software, intelligent machines, event stream processing applications, and operational intelligence platforms. As a result, the demand for analytics has moved outside specialty IT-based communities and is now headed up primarily by individual business units.
As you become a data head, your job is to demonstrate leadership in asking questions about the data used on a project. I am talking about the underlying raw data from which all the statistics are calculated, machine learning models built, or dashboard visualizations created.
The data types dictate the analysis method. If the raw data is wrong, then your models, visualizations, machine learning, and statistics are wrong. Garbage in, garbage out.
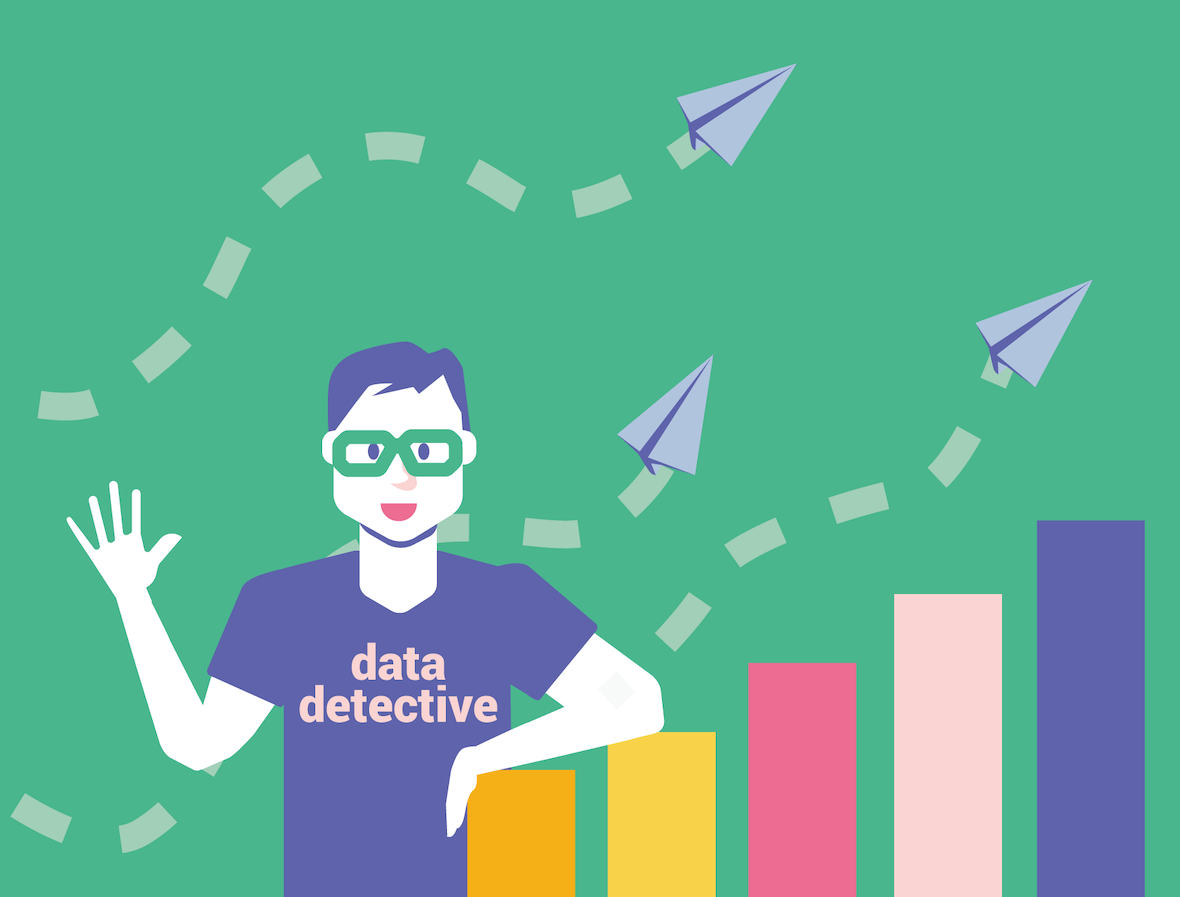
Here are a few questions that should be able to help you to argue with data, these questions open opportunities for follow-up points and discussions.
- This question intends to create a sense of openness and build trust in the results. It doesn't require mathematical or statical knowledge to answer.
- This question looks to understand precisely where the data originated and if there are issues surrounding its origin: Internet data, third-party vendor, or external data. You're looking to know if the data is reliable.
- This question concerns ethical issues with data collection and what is allowed and what is not. There are two basic data collection methods observational and experimental.
- Observational data is collected passively. (most business)
- Experimental data is collected under certain conditions to my twin integrity and to avoid confusion. Experimental data is the gold standard.
- You must work with the data you have while also meditating on its ability to drive business decisions. Some companies and departments have the resources to follow up on promising observational data with solar experiments. And yet, other business problems do not easily lend themselves to experiment.
- This doesn't require an explanation—play detective.
- Data cannot make sure anything and everything. Data measure something, and you should be truthful and honest about it.
- This question is about patterns that were unknown or not so obvious at first glance. Aha moments.
- Findings can also help us to redefine or formulate a new problem or opportunity valuable to the team or organization.
- It's all about a clearer path forward to solve a specific problem.
That's the primary intention of this blog post to present you with questions you should ask and the various issues these questions cover.
Dig deeper into the issues surrounding your data, and you might come up with additional questions. Share these questions with the rest of your team, so you are all aligned. They can demonstrate their ability to go through data by setting an example and asking it tough questions on an ongoing basis.
Most businesses don't argue with their data. Instead, they have a culture of acceptance. The effect of these is a slow burn where the data project continues to fail without essential questions being asked during the project.
Data start somewhere, and we shouldn't take for granted its origins. A good data team does not follow a linear path but a meandering one, adapting to discovery in the data.